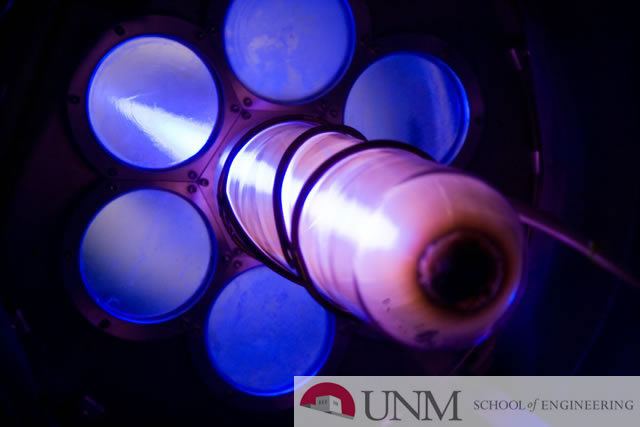
Electrical and Computer Engineering ETDs
Publication Date
Fall 11-11-2020
Abstract
The advances of multi-access edge computing (MEC) have paved the way for the integration of the MEC servers, as intelligent entities into the Internet of Things (IoT) environment as well as into the 5G radio access networks. In this thesis, a novel artificial intelligence-based MEC servers’ activation mechanism is proposed, by adopting the principles of Reinforcement Learning (RL) and Bayesian Reasoning. The considered problem enables the MEC servers’ activation decision-making, aiming at enhancing the reputation of the overall MEC system, as well as considering the total computing costs to serve efficiently the users’ computing demands, guaranteeing at the same time their Quality of Experience (QoE) prerequisites satisfaction. Each MEC server decides in an autonomous manner whether it will be activated or remain in sleep mode by utilizing the theory of Bayesian Learning Automata (BLA). A human-driven peer-review-based evaluation of the edge computing system’s provided services is also introduced based on the concept of Bayesian Truth Serum (BTS), which supports the development of a reputation mechanism regarding the MEC servers' provided services. The intelligent MEC servers’ autonomous decisions’ satisfaction is captured via a holistic utility function, which they aim to maximize in a distributed manner. Finally, detailed numerical results obtained via modeling and simulation, highlight the key operation features and superiority of the proposed framework.
Keywords
Mobile Edge Computing, Bayesian Learning Automata, Bayesian Truth Serum, Multi-Access edge computing, Artificial Intelligent Server Activation
Document Type
Thesis
Language
English
Degree Name
Computer Engineering
Level of Degree
Masters
Department Name
Electrical and Computer Engineering
First Committee Member (Chair)
Eirini Tsiropoulou
Second Committee Member
Jim Plusquellic
Third Committee Member
Michael Devetsikiotis
Recommended Citation
Lebien, Sean. "Incentivization in Mobile Edge Computing using a Full Bayesian Approach." (2020). https://digitalrepository.unm.edu/ece_etds/496
Included in
Digital Communications and Networking Commons, Electrical and Computer Engineering Commons