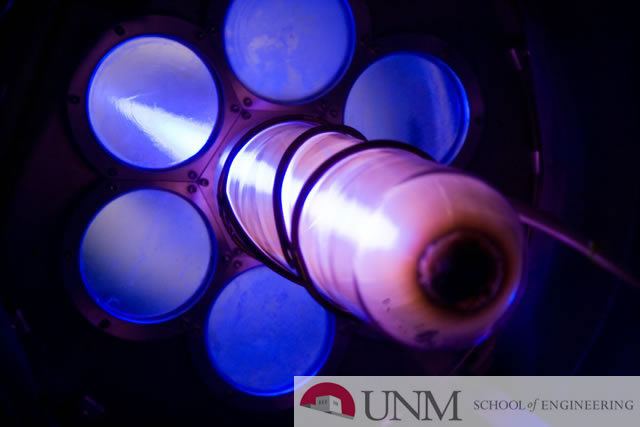
Electrical and Computer Engineering ETDs
Publication Date
Fall 11-15-2016
Abstract
Mental disorders such as schizophrenia (SZ), bipolar (BD), and major depression disorders (MDD) can cause severe symptoms and life disruption. They share some symptoms, which can pose a major clinical challenge to their differentiation. Objective biomarkers based on neuroimaging may help to improve diagnostic accuracy and facilitate optimal treatment for patients. Over the last decades, non-invasive in-vivo neuroimaging techniques such as magnetic resonance imaging (MRI) have been increasingly applied to measure structure and function in human brains. With functional MRI (fMRI) or structural MRI (sMRI), studies have identified neurophysiological deficits in patients’ brain from different perspective. Functional connectivity (FC) analysis is an approach that measures functional integration in brains. By assessing the temporal coherence of the hemodynamic activity among brain regions, FC is considered capable of characterizing the large-scale integrity of neural activity.
In this work, we present two data analysis frameworks for biomarker detection on brain imaging with FC, 1) graph analysis of FC and 2) multimodal fusion analysis, to better understand the human brain. Graph analysis reveals the interaction among brain regions based on graph theory, while the multimodal fusion framework enables us to utilize the strength of different imaging modalities through joint analysis. Four applications related to FC using these frameworks were developed. First, FC was estimated using a model-based approach, and revealed altered the small-world network structure in SZ. Secondly, we applied graph analysis on functional network connectivity (FNC) to differentiate BD and MDD during resting-state. Thirdly, two functional measures, FNC and fractional amplitude of low frequency fluctuations (fALFF), were spatially overlaid to compare the FC and spatial alterations in SZ. And finally, we utilized a multimodal fusion analysis framework, multi-set canonical correlation analysis + joint independent component analysis (mCCA+jICA) to link functional and structural abnormalities in BD and MDD. We also evaluated the accuracy of predictive diagnosis through classifiers generated on the selected features. In summary, via the two frameworks, our work has made several contributions to advance FC analysis, which improves our understanding of underlying brain function and structure, and our findings may be ultimately useful for the development of biomarkers of mental disease.
Keywords
Functional connectivity, multimodal fusion, graph analysis, neuroimaging, biomarker, mental disorders
Document Type
Dissertation
Language
English
Degree Name
Computer Engineering
Level of Degree
Doctoral
Department Name
Electrical and Computer Engineering
First Committee Member (Chair)
Vince D. Calhoun
Second Committee Member
Vincent P. Clark
Third Committee Member
Marios S. Pattichis
Fourth Committee Member
Jing Sui
Recommended Citation
He, Hao. "SEARCHING NEUROIMAGING BIOMARKERS IN MENTAL DISORDERS WITH GRAPH AND MULTIMODAL FUSION ANALYSIS OF FUNCTIONAL CONNECTIVITY." (2016). https://digitalrepository.unm.edu/ece_etds/303
Included in
Electrical and Computer Engineering Commons, Psychiatric and Mental Health Commons, Statistics and Probability Commons