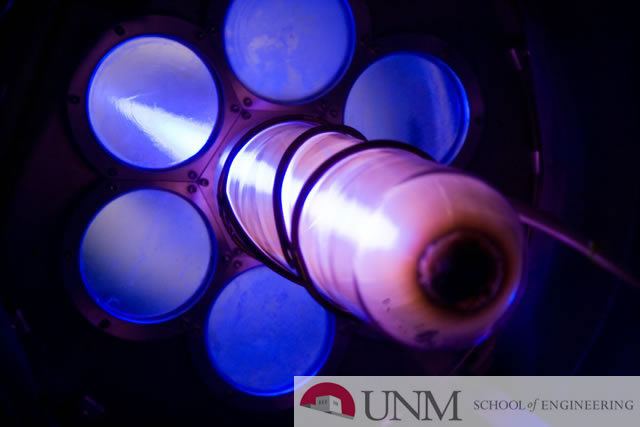
Electrical and Computer Engineering ETDs
Publication Date
9-12-2014
Abstract
The human brain is one of the most complex systems known to the mankind. Over the past 3500 years, mankind has constantly investigated this remarkable system in order to understand its structure and function. Emerging of neuroimaging techniques such as functional magnetic resonance imaging (fMRI) have opened a non-invasive in-vivo window into brain function. Moreover, fMRI has made it possible to study brain disorders such as schizophrenia from a different angle unknown to researchers before. Human brain function can be divided into two categories: functional segregation and integration. It is well-understood that each region in the brain is specialized in certain cognitive or motor tasks. The information processed in these specialized regions in different temporal and spatial scales must be integrated in order to form a unified cognition or behavior. One way to assess functional integration is by measuring functional connectivity (FC) among specialized regions in the brain. Recently, there is growing interest in studying the FC among brain functional networks. This type of connectivity, which can be considered as a higher level of FC, is termed functional network connectivity (FNC) and measures the statistical dependencies among brain functional networks. Each functional network may consist of multiple remote brain regions. Four studies related to FNC are presented in this work. First FNC is compared during the resting-state and auditory oddball task (AOD). Most previous FNC studies have been focused on either resting-state or task-based data but have not directly compared these two. Secondly we propose an automatic diagnosis framework based on resting-state FNC features for mental disorders such as schizophrenia. Then, we investigate the proper preprocessing for fMRI time-series in order to conduct FNC studies. Specifically the impact of autocorrelated time-series on FNC will be comprehensively assessed in theory, simulation and real fMRI data. At the end, the notion of autoconnectivity as a new perspective on human brain functionality will be proposed. It will be shown that autoconnectivity is cognitive-state and mental-state dependent and we discuss how this source of information, previously believed to originate from physical and physiological noise, can be used to discriminate schizophrenia patients with high accuracy.
Keywords
Functional Network Connectivity, Machine Learning, Neuroimaging, Human Brain
Document Type
Dissertation
Language
English
Degree Name
Electrical Engineering
Level of Degree
Doctoral
Department Name
Electrical and Computer Engineering
First Committee Member (Chair)
Caudell, Thomas
Second Committee Member
Erhardt, Erik
Third Committee Member
Pattichis, Marios
Fourth Committee Member
Calhoun, Vince
Recommended Citation
Arbabshirani, Mohammad. "FUNCTIONAL NETWORK CONNECTIVITY IN HUMAN BRAIN AND ITS APPLICATIONS IN AUTOMATIC DIAGNOSIS OF BRAIN DISORDERS." (2014). https://digitalrepository.unm.edu/ece_etds/18