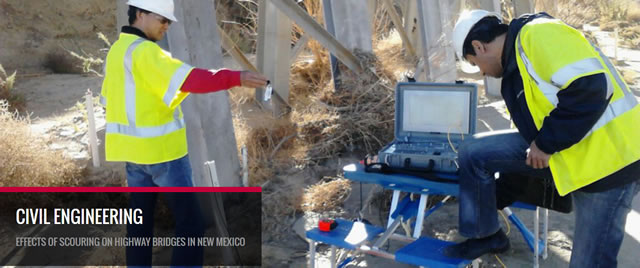
Civil Engineering ETDs
Publication Date
2-1-2016
Abstract
Traffic crashes have resulted in significant cost to society in terms of life and economic losses, and comprehensive examination of crash injury outcome patterns is of practical importance. By inferring the parameters of interest from prior information and studied datasets, Bayesian models are efficient methods in data analysis with more accurate results, but their applications in traffic safety studies are still limited. By examining the driver injury severity patterns, this research is proposed to systematically examine the applicability of Bayesian methods in traffic crash driver injury severity prediction in traffic crashes. In this study, three types of Bayesian models are defined: hierarchical Bayesian regression model, Bayesian non-regression model and knowledge-based Bayesian non-parametric model, and a conceptual framework is developed for selecting the appropriate Bayesian model based on discrete research purposes. Five Bayesian models are applied accordingly to test their effectiveness in traffic crash driver injury severity prediction and variable impact estimation: hierarchical Bayesian binary logit model, hierarchical Bayesian ordered logit model, hierarchical Bayesian random intercept model with cross-level interactions, multinomial logit (MNL)-Bayesian Network (BN) model, and decision table/na\xefve Bayes (DTNB) model. A complete dataset containing all crashes occurring on New Mexico roadways in 2010 and 2011 is used for model analyses. The studied dataset is composed of three major sub-datasets: crash dataset, vehicle dataset and driver dataset, and all included variables are therefore divided into two hierarchical levels accordingly: crash-level variables and vehicle/driver variables. From all these five models, the model performance and analysis results have shown promising performance on injury severity prediction and variable influence analysis, and these results underscore the heterogeneous impacts of these significant variables on driver injury severity outcomes. The performances of these models are also compared among these methods or with traditional traffic safety models. With the analyzed results, tentative suggestions regarding countermeasures and further research efforts to reduce crash injury severity are proposed. The research results enhance the understandings of the applicability of Bayesian methods in traffic safety analysis and the mechanisms of crash injury severity outcomes, and provide beneficial inference to improve safety performance of the transportation system.
Keywords
Bayesian Methods, Driver Injury Severity, Traffic Crash Data, Traffic Safety
Document Type
Dissertation
Language
English
Degree Name
Civil Engineering
Level of Degree
Doctoral
Department Name
Civil Engineering
First Committee Member (Chair)
Halter, Susan Bogus
Second Committee Member
Yang, Yin
Third Committee Member
Tarefder, Rafiqul A.
Recommended Citation
Chen, Cong. "DATA-DRIVEN BAYESIAN METHOD-BASED TRAFFIC CRASH DRIVER INJURY SEVERITY FORMULATION, ANALYSIS, AND INFERENCE." (2016). https://digitalrepository.unm.edu/ce_etds/19