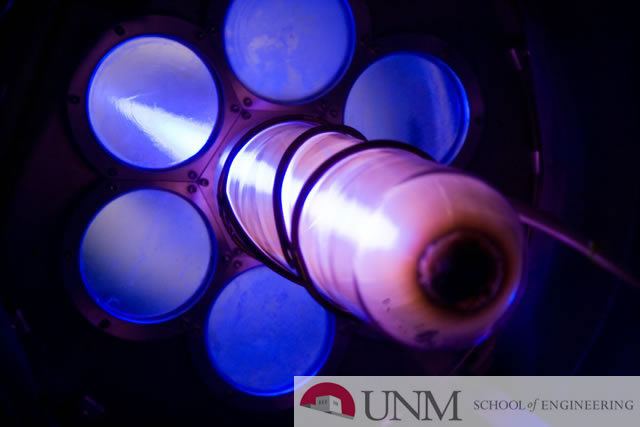
Electrical and Computer Engineering ETDs
Publication Date
6-24-2015
Abstract
Skin cancer is the most common cancer in the United States with over 3.5M annual cases. Statistics from the Americans Cancer Society indicate that 20% of the American population will develop this disease during their lifetime. Presently, visual inspection by a dermatologist has good sensitivity (>90%) but poor specificity (<10%), especially for melanoma conditions, which is the most dangerous type of skin cancer with a five-year survival rate between 16-62%. Over the past few decades, several studies have evaluated the use of infrared imaging to diagnose skin cancer. Here we use dynamic thermal imaging (DTI) to demonstrate a rapid, accurate and non-invasive imaging and processing technique to diagnose melanoma and non-melanoma skin cancer lesions. In DTI, the suspicious lesion is cooled down and the thermal recovery of the skin is monitored with an infrared camera. The proposed algorithm exploits the intrinsic order present in the time evolution of the thermal recoveries of the skin of human subjects to diagnose the malignancy and it achieves outstanding performance for discriminating between benign and malignant skin lesions. In this dissertation we propose a stochastic parametric representation of the thermal recovery curve, which is extracted from a heat equation. The statistics of the random parameters associated with the proposed stochastic model are estimated from measured thermal recovery curves of subjects with known condition. The stochastic model is, in turn, utilized to derive an analytical autocorrelation function (ACF) of the stochastic recovery curves. The analytical ACF is utilized in the context of continuous-time detection theory in order to define an optimal statistical decision rule such that the sensitivity of the algorithm is guaranteed to be at a maximum for every prescribed false-alarm probability. The proposed algorithm was tested in a pilot study including 140 human subjects and we have demonstrated sensitivity in excess of 99% for a prescribed false-alarm probability of 1% (specificity in excess of 99%) for detection of skin cancer. To the best of our knowledge, this is the highest reported accuracy for any non-invasive skin cancer diagnosis method. The proposed algorithm is studied in details for different patient permutations demonstrating robustness in maximizing the probability of detecting those subjects with malignant condition. Moreover, the proposed method is further generalized to include thermal recovery curves of the tissue that surrounds the suspicious lesion as a local reference. Such a local reference permits the compensation of any possible anomalous behavior in the lesion thermal recovery, which, in turn, improves both the theoretical and empirical performance of the method. As a final contribution, we develop a novel edge-detection algorithm--specifically targeted for multispectral (MS) and hyperspectral (HS) imagery--which performs l edge detection based solely on spectral (color) information. More precisely, this algorithm fuses the process of detecting edges through ratios of pixels with critical information resulting from spectral classification of the very image whose edges are to be identified. This algorithm is tested in multicolor (spectral) imagery achieving superior results as compared with other alternatives. The edge-detection algorithm is subsequently utilized in the skin-cancer detection context to define the lesion boundary from a visible color image by exploiting the color contrast between the pigmented tissue and the surrounding skin. With this automated lesion selection, we develop a method to extract spatial features equivalent to those utilized by the dermatologists in diagnosing malignant conditions. These spatial features are fused with the temporal features, obtained from the thermal-recovery method, to yield a spatio-temporal method for skin-cancer detection. While providing a rigorous mathematical foundation for the viability of the dynamic thermal recovery approach for skin-cancer detection, the research completed in this dissertation also provides the first reliable, accurate and non-invasive diagnosis method for preliminary skin-cancer detection. This dissertation, therefore, paves the way for future clinical studies to produce new skin-cancer diagnosis practices that minimize the need for unnecessary biopsies without sacrificing reliability.
Keywords
Detection theory, Skin-cancer detection, Statistical inference, Karhunen-Loeve expansion, Dynamic infrared imaging
Sponsors
This work was partially supported by the Department of Energy NNSA (NA-22) DE-NA0002494 award and internal funds from Skinfrared, LLC. Some of the patient data collection was supported by a SBIR Phase I award #1315372 from the National Science Foundation. S. E. Godoy was also supported in part by the Comisio\u0301n Nacional de Investigacio\u0301n Cienti\u0301fica y Tecnolo\u0301gica (CONICYT), Chile, the New Mexico Cancer Nanotechnology Training Center (CNTC) fellowship, University of New Mexico and the University of Concepcio\u0301n, Chile.
Document Type
Dissertation
Language
English
Degree Name
Electrical Engineering
Level of Degree
Doctoral
Department Name
Electrical and Computer Engineering
First Committee Member (Chair)
Krishna, Sanjay
Second Committee Member
Calhoun, Vincent
Third Committee Member
Padilla, R. Steven
Recommended Citation
Godoy, Sebastian. "Communication-theoretic Approach for Skin Cancer Detection using Dynamic Thermal Imaging." (2015). https://digitalrepository.unm.edu/ece_etds/99