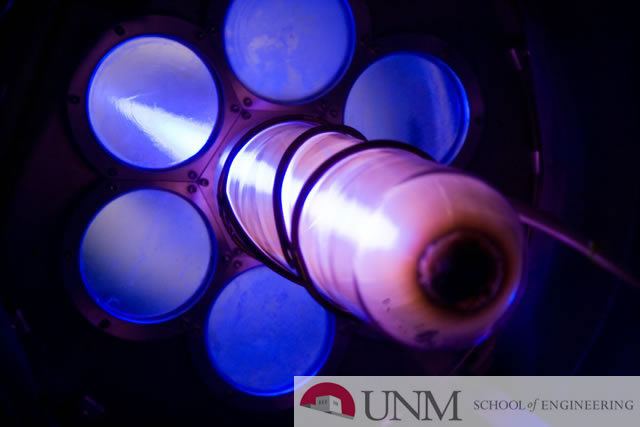
Electrical and Computer Engineering ETDs
Publication Date
2-13-2014
Abstract
Mental disorders are diagnosed on the basis of reported symptoms and externally observed clinical signs. Nonetheless, these cannot be evaluated by means of clinical tests. This is the case for schizophrenia, a complex disease characterized by perturbations in language, perception, thinking, social relationships and will that affects about 1% of the U.S. population. Besides the absence of an objective assessment of symptoms to diagnose schizophrenia, not even a set of symptoms that uniquely characterize this disorder have been found. Given the absence of a biologically-based diagnosis of schizophrenia, several studies have used different brain imaging techniques in an attempt to characterize the biological abnormalities found on patients. One of those techniques is functional magnetic resonance imaging (fMRI), a non-invasive technique that captures brain images that reflect neuronal activity. While fMRI studies have been able to provide significant information about schizophrenia, the acquired data present some technical challenges. FMRI characterizes the dynamics of brain activity in time for several brain volumetric elements (voxels), thus generating massive amounts of data. On the other hand, fMRI studies acquire images from tens or hundreds of subjects, the rate between the data dimensionality and the sample size being very high. One way of dealing with this issue is to use univariate approaches to analyze the data, i.e., analyze each voxel individually. However, such approaches neglect the spatial correlation in the data. Machine learning algorithms can be used to do a multivariate analysis of fMRI data and predict a condition of interest. In addition, the contribution of the analyzed features (in this case voxels) to the learning task can be estimated. Nonetheless, these algorithms' performance is also precluded by the high dimensionality of fMRI data, making whole-brain approaches prone to poorly fit the data. For this reason, some studies restricted their analyses to sets of voxels within certain regions of interest (ROIs). While such approaches are able to solve the dimensionality problem, they do it at the expense of losing information from other potentially informative regions. Furthermore, these studies perform an interpretation of the results at a voxel level and not at a brain region level, which could potentially be richer and more meaningful. Under the assumption that activation is sparsely distributed across the brain, methods that are capable of performing a sparse region selection would be able to address the dimensionality problem and provide a better interpretation of brain activation patterns. Such functionality can be attained by using multiple kernel learning approaches. This dissertation proposes a machine learning framework based on a multiple-kernel data representation to distinguish groups of schizophrenia patients from healthy controls using fMRI data, the activation patterns of each brain region being characterized by a kernel. This approach is capable of performing a sparse selection of informative regions and it is flexible enough to exploit linear or nonlinear relationships among the voxels within them. Two algorithms that follow this framework are presented: recursive composite kernels (RCK) and v-multiple kernel learning (v-MKL). This work evaluates these algorithms in terms of their prediction performance, the validity of the brain regions that are deemed relevant and their capacity to analyze diverse data sources.
Keywords
multiple kernel learning, schizophrenia, machine learning, fMRI, medical imaging, SVM
Document Type
Dissertation
Language
English
Degree Name
Computer Engineering
Level of Degree
Doctoral
Department Name
Electrical and Computer Engineering
First Committee Member (Chair)
Clark, Vince P.
Second Committee Member
Pattichis, Marios
Recommended Citation
Castro Witting, Eduardo Jose. "Application of Multiple Kernel Learning on Brain Imaging for Mental Illness Characterization." (2014). https://digitalrepository.unm.edu/ece_etds/46