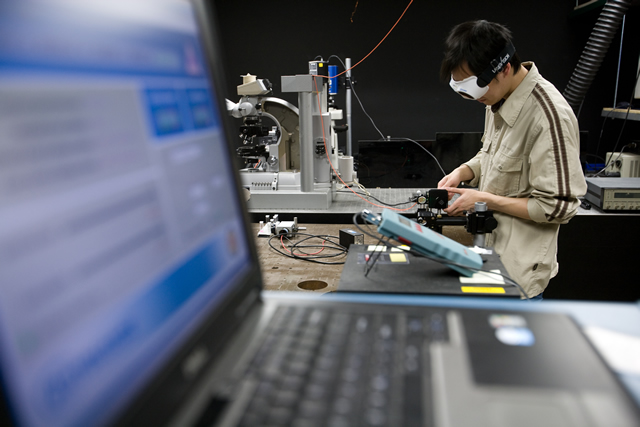
Computer Science ETDs
Publication Date
5-1-2010
Abstract
Bayesian belief aggregation is the process of forming a consensus model from the probabilistic beliefs of multiple individuals. Preference aggregation attempts to find an optimal solution for a population considering each individual's beliefs, desires and objectives. Belief and preference aggregation approaches that form a single consensus average away any diversity in a population. In the process they may fail to uphold a set of mathematical properties for rational aggregation defined by social choice theorists. This dissertation introduces a new aggregation approach that maintains the diversity of a population and allows the competitive aspects of a situation to emerge, enabling game theoretic analysis in large populations of decision-makers. Each individual's beliefs and preferences are represented by a Bayesian network. Based on the result of inference on the networks, a population is separated into collectives whose members agree on the relatively likelihood or desirability of the possible outcomes of a situation. An aggregate for each collective can then be computed such that the aggregate upholds the rationality properties. Game theoretic analysis is then applied using 'super-agents' that represent each collective as the game players. In this manner, the set of Pareto optimal and Nash equilibrium solutions can be found, even in situations that cause single consensus models to return non-Pareto or otherwise 'irrational' solutions.
Language
English
Keywords
Decision-making, Bayesian reasoning, Social choice theory, Probabilistic reasoning, Bayesian belief aggregation, game theory, diversity, artificial intelligence, social decision-making
Document Type
Dissertation
Degree Name
Computer Science
Level of Degree
Doctoral
Department Name
Department of Computer Science
First Committee Member (Chair)
Kniss, Joe
Second Committee Member
Moses, Melanie
Third Committee Member
Ross, Tim
Fourth Committee Member
Stern, Carl
Recommended Citation
Greene, Kshanti. "Collective belief models for representing consensus and divergence in communities of Bayesian decision-makers." (2010). https://digitalrepository.unm.edu/cs_etds/8